Designer Demographics and Statistics 2024 : Number Of Designers In The US
Table Of Content

This means that we only observe every treatment once in eachblock. When we want to infer if the amount of noise explained by adding Irrigation or Fertilizer is sufficiently large to justify their inclusion into the model, we compare the sum-of-squares value to the RSS but now we have to use the appropriate pool. In this case we see that we have insufficient evidence to conclude that the observed difference between the Irrigation levels could not be due to random chance.
General balanced designs (t-designs)
Criteria for good data visualization, according to design and statistics - Quartz
Criteria for good data visualization, according to design and statistics.
Posted: Sun, 12 Jan 2020 08:00:00 GMT [source]
The cells in the matrix have indices that match the X1, X2 combinations above. The final step in the blocking process is allocating your observations into different treatment groups. All you have to do is go through your blocks one by one and randomly assign observations from each block to treatment groups in a way such that each treatment group gets a similar number of observations from each block.
What is a Randomized Block Experiment?
If this assumption is violated, randomized block ANOVA should not performed. One possible alternative is to treat it like a factorial ANOVA where the independent variables are allowed to interact with each other. The dataset oatvar in the faraway library contains information about an experiment on eight different varieties of oats. Within each block, the researchers created eight plots and randomly assigned a variety to a plot. This type of design is called a Randomized Complete Block Design (RCBD) because each block contains all possible levels of the factor of primary interest. While it is true randomized block design could be more powerful than single-factor between-subjects randomized design, this comes with an important condition.
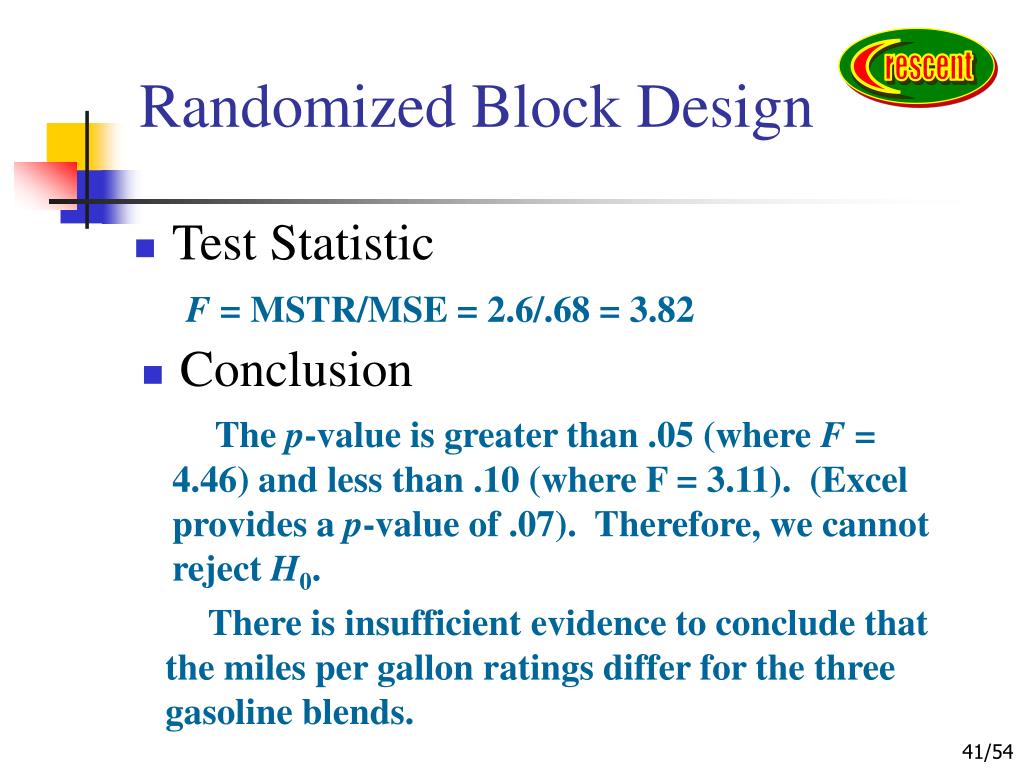
Blocking in experimental design
As an example, imagine you were running a study to test two different brands of soccer cleats to determine whether soccer players run faster in one type of cleats or the other. Further, imagine that some of the soccer players you are testing your cleats on only have grass fields available to them and others only have artificial grass or turf fields available to them. Now, say you have reason to believe that athletes tend to run 10% faster on turf fields than grass fields. Statology is a site that makes learning statistics easy by explaining topics in simple and straightforward ways. Our team of writers have over 40 years of experience in the fields of Machine Learning, AI and Statistics. In the most basic form, we assume that we do not have replicateswithin a block.

If the blocks aren't homogeneous, their variability will not be less than that of the entire sample. In that situation, randomized block design can decreases the statistical power and thus be worse than a simple single-factor between-subjects randomized design. Again, your best bet on finding an optimal number of blocks is from theoretical and/or empirical evidences. Once the participants are placed into blocks based on the blocking variable, we would carry out the experiment to examine the effect of cell phone use (yes vs. no) on driving ability.
The first step of implementing blocking is deciding what variables you need to balance across your treatment groups. Here are some examples of what your blocking factor might look like. First the individual observational units are split into blocks of observational units that have similar values for the key variables that you want to balance over. After that, the observational units from each block are evenly allocated into treatment groups in a way such that each treatment group is allocated similar numbers of observational units from each block. The key element is that each treatment level or treatment combination appears in each block (forming complete blocks), and is assigned at random within each block.
But the variation between blocks has to be incorporated into the model and will be partitioned out of the Error Mean squares of the CRD, resulting in a smaller MSE for testing hypotheses about treatments. By placing the individuals into blocks, the relationship between the new diet and weight loss became more clear since we were able to control for the nuisance variable of gender. This kind of design is used to minimize the effects of systematic error.
Those in each block will be randomly assigned into either treatment conditions of the independent variable, cell phone use (yes vs. no). As we carry out the study, participants' driving ability will be assessed. We can determine whether cell phone use has an effet on driving ability after controlling for driving experience. In randomized block design, the control technique is done through the design itself.
Accurate autocorrelation modeling substantially improves fMRI reliability - Nature.com
Accurate autocorrelation modeling substantially improves fMRI reliability.
Posted: Thu, 21 Mar 2019 07:00:00 GMT [source]
Identify nuisance variables
First we discuss what blocking is and what its main benefits are. After that, we discuss when you should use blocking in your experimental design. Finally, we walk through the steps that you need to take in order to implement blocking in your own experimental design. Here, the number of rows to be specified is our block size (and number of treatment levels), which yields a random assignment from Block 1.
The use of blocking in experimental design has an evolving history that spans multiple disciplines. The foundational concepts of blocking date back to the early 20th century with statisticians like Ronald A. Fisher. His work in developing analysis of variance (ANOVA) set the groundwork for grouping experimental units to control for extraneous variables. Note that blocking is a special way to design an experiment, or a special“flavor” of randomization.
If batches are randomly selected then they are random effects. That is , if the experiment was repeated, a new sample of i batches would be selected,d yielding new values for \(\rho_1, \rho_2,...,\rho_i\) then. Next we can do the appropriate analysis for the fertilizer, recognizing that all the p-values for the plot effects are nonsense and should be ignored.
Because the specific details of how blocking is implemented can vary a lot from one experiment to another. For that reason, we will start off our discussion of blocking by focusing on the main goal of blocking and leave the specific implementation details for later. In this article we tell you everything you need to know about blocking in experimental design.
Graphic design and marketing are heavily linked to each other, with the former often determining the latter’s success. To help pay the bills, we’ll often (but not always) set up affiliate relationships with the top providers after selecting our favorites. There are plenty of high-paying companies we’ve turned down because we didn’t like their product. See how designer racial and ethnic diversity trended since 2010 according to the United States Census Bureau data.
Fortunately in this case, we don’t care about the blocking variable and including it in the model was simply guarding us in case there was a difference, but I wasn’t interested in estimating it. If the only covariate we care about is the most deeply nested effect, then we can do the usual analysis and recognize the p-value for the blocking variable is nonsense, and we don’t care about it. An agricultural field study has three fields in which the researchers will evaluate the quality of three different varieties of barley. Due to how they harvest the barley, we can only create a maximum of three plots in each field. In this example we will block on field since there might be differences in soil type, drainage, etc from field to field.
Comments
Post a Comment